Measuring the immeasurable : an experiment for a machine to map low-level features to high-level semantic representation of architectural space using a single view photo
Author(s)
Liu, Yuxuan,S. M.Massachusetts Institute of Technology.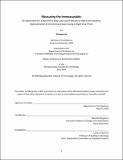
Download1193319937-MIT.pdf (11.75Mb)
Alternative title
Experiment for a machine to map low-level features to high-level semantic representation of architectural space using a single view photo
Other Contributors
Massachusetts Institute of Technology. Department of Architecture.
Advisor
Takehiko Nagakura.
Terms of use
Metadata
Show full item recordAbstract
Constructing experience is an important part in architectural design, and experience-oriented design is highly dependent on an architect's subjective understanding of space. Although design and computer science have become more closely integrated in recent years, commercialized computer-aided design (CAD) systems are still only able to substitute labor-intensive processes, such as auto-generation of architectural drawings from digital models but offer little support in actual design. The human-centric design process is still not yet substitutable by non-human system, due to non-human system's lack of the ability to understand space subjectively. This thesis' focus is on developing machine awareness of space. The research method is on translating human-level spatial awareness represented by adjectives in language to a non-human system through single view photo based on a data-driven machine learning method. As humans show general consistency in subjective understanding of space, experiments are designed and published on crowd-sourcing platform Amazon Mechanical Turk (AMT) to match architectural space represented in single shot photo with subjective understanding of it represented by adjectives in language. These collected data will assist the development of a machine system. The completed proposed system employs techniques such as 3D geometry reconstruction from a single image, surface simplification, scene attributes extraction, color vividness extraction in order to understand low-level features of space. This low-level understanding is then translated to the likeliest high-level, text-based understanding by the machine system based on a data-driven machine learning method. As in this thesis, adjectives are regarded as the signal of human's subjective understanding of space, it also explores the subjective mental process in people's mind of decoding the space's nature through text-based representation. Eventually, as the machine system gains the ability of understanding space from both low-levels features and high-level features, it potentially could help to build a hybrid design system that designs by form and design by experience.
Description
Thesis: S.M., Massachusetts Institute of Technology, Department of Architecture, May, 2020 Cataloged from the official PDF of thesis. Includes bibliographical references (pages 73-74).
Date issued
2020Department
Massachusetts Institute of Technology. Department of ArchitecturePublisher
Massachusetts Institute of Technology
Keywords
Architecture.